In Press
Hello!! I'm Brian Tang.
4th year CSE Ph.D. Candidate, University of Michigan.
I create software systems for privacy using AI.
I'm a member of the RTCL advised by Kang G. Shin. I also collaborate with SpiLab and Florian Schaub.
In undergrad, I worked on machine learning security & privacy in WI-PI with Kassem Fawaz, Varun Chandrasekaran, and Somesh Jha.
Please contact me via bjaytang@umich.edu.
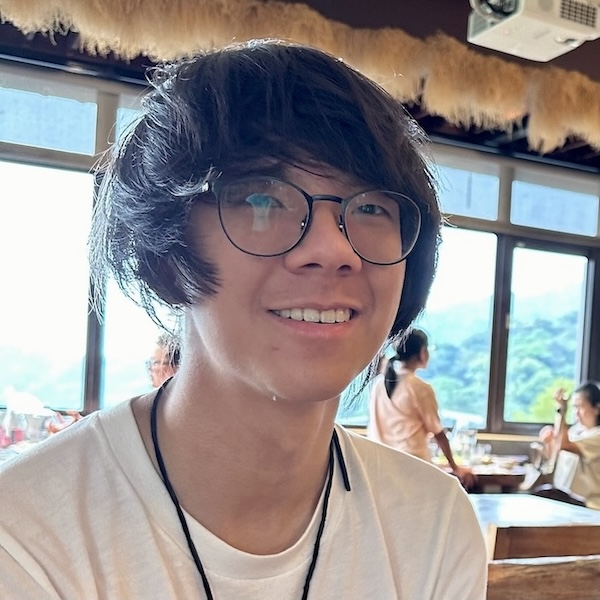